Abstracts
Arash Afraz
Navigating perceptual space with neural perturbations
Local perturbation of neural activity in high-level visual cortical areas alters visual perception. Quantitative characterization of these perceptual alterations holds the key to understanding the mapping between patterns of neuronal activity and elements of perception. The complexity and subjectivity of these perceptual alterations makes them difficult to study. I introduce a new experimental approach, “Perceptography”, to develop “pictures” of the subjective experience induced by optogenetic cortical stimulation in the inferior temporal cortex of macaque monkeys.
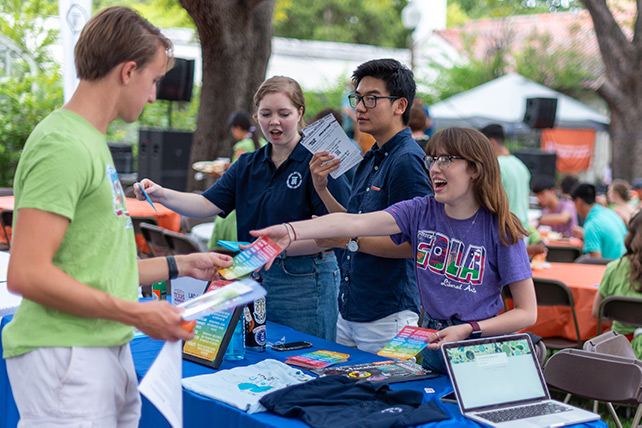
Rowan Candy
The statistics of the natural visual experience selected by infants
Human infants learn to interact with the world over the first months after birth. They use their ocular motor responses to select structure from the earliest ages in the dynamic three-dimensional environment, even before they begin to reach and move through their surroundings. Their immature visual function must support this active development by providing the sequential diet of information required for learning everything from basic motor skills to high level cognition. Here I will review our recent work designed to reveal the low-level statistics of this early visual input. Using head-mounted cameras and binocular eye-tracking, we have characterized the structure selected by infants from 2-15 months during natural unrestricted activities..
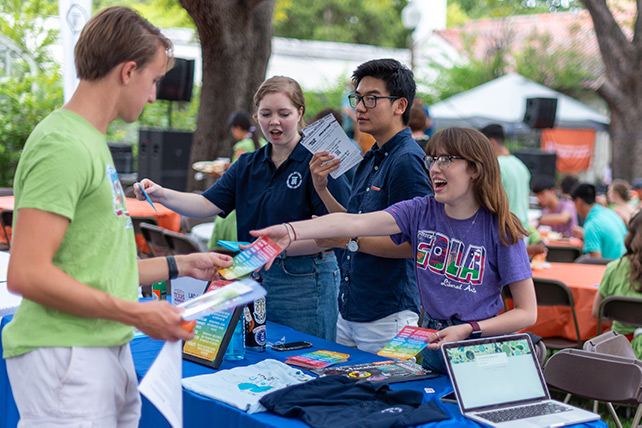
SueYeon Chung
Computing with Neural Manifolds: Towards a Multi-Scale Understanding of Biological and Artificial Neural Networks
Recent breakthroughs in experimental neuroscience and machine learning have opened new frontiers in understanding the computational principles governing neural circuits and artificial neural networks (ANNs). Both biological and artificial systems exhibit an astonishing degree of orchestrated information processing capabilities across multiple scales - from the microscopic responses of individual neurons to the emergent macroscopic phenomena of cognition and task functions. At the mesoscopic scale, the structures of neuron population activities manifest themselves as neural representations. Neural computation can be viewed as a series of transformations of these representations through various processing stages of the brain. The primary focus of my lab's research is to develop theories of neural representations that describe the principles of neural coding and, importantly, capture the complex structure of real data from both biological and artificial systems.
In this talk, I will present three related approaches that leverage techniques from statistical physics, machine learning, and geometry to study the multi-scale nature of neural computation. First, I will introduce new statistical mechanical theories that connect geometric structures that arise from neural responses (i.e., neural manifolds) to the efficiency of neural representations in implementing a task. Second, I will employ these theories to analyze how these representations evolve across scales, shaped by the properties of single neurons and the transformations across distinct brain regions. Finally, I will demonstrate how insights from the theories of neural representations can elucidate why certain ANN models better predict neural data, facilitating model comparison and selection.
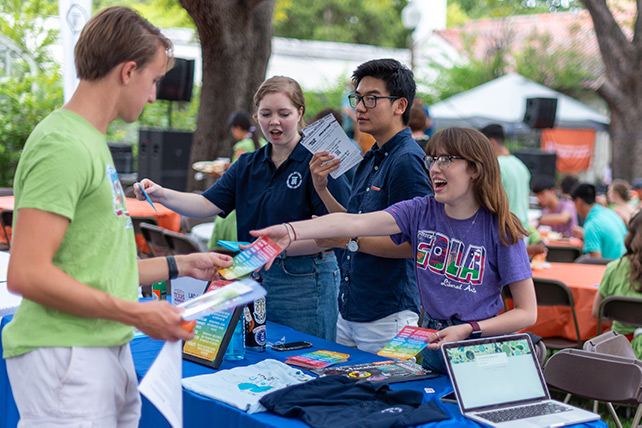
Mark Churchland
Neurobiology of flexible deductive reasoning
Primates can solve novel problems through logical and stepwise reasoning. No two real-world situations are the same, and how one ‘figures out’ a solution may be similarly variable. Studying reasoning has thus been challenging. How should one investigate the neural basis of internal events whose timing and nature are uncertain, and are unlikely to ever unfold the same way twice? To meet this challenge, we used large-scale Neuropixels-probe recordings and a novel task where monkeys apply abstract knowledge to determine the correct ordering of stimuli on the screen. Neural activity in prefrontal cortex (but not in motor cortex) reflected the sequential ‘figuring out’ of a solution. The set of internal steps, and their timing, were different on every trial. For example, the animal might sometimes figure out the last element first, and work backwards. On other trials they might use the opposite approach. In some ways neural activity was complex: a set of multiple choices could be made in any order, involved physical locations that could be anywhere on the screen, and had to respect a rule that varied on every trial. Yet in some sense neural activity was simple, and more step-wise than one might expect. At any specific moment, the animal was engaged in a single internal choice, governed by the current rule and precisely one stimulus. He then committed that choice to memory, and moved on to the next decision. These events were entirely internal, and occurred before a go cue was given and choices were rendered through action. These results show that step-like reasoning is used by monkeys to solve problems, and affords great flexibility. The same neural ‘strategy’ can unfold very differently on different trials, yet still solve the problem at hand successfully.
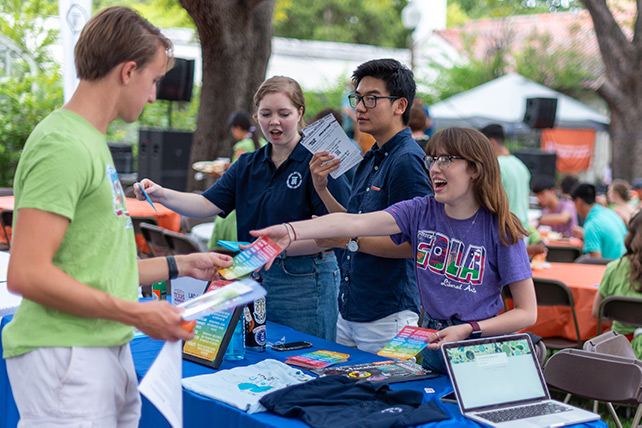
Sven Dickinson
Symmetry in Human and Computer Vision: a Case Study in Scene Perception
Symmetry is one of the most ubiquitous regularities in our natural world. For 100 years, human vision researchers have studied how the human vision system has evolved to exploit this powerful regularity for perceptual grouping. In the computer vision community, early (pre-deep learning) researchers also exploited symmetry, and developed elegant representations for symmetry in support of segmentation, grouping, 3-D reconstruction, and object recognition. In the first part of the talk, I will review our research that draws on a symmetry-based representation in computer vision to identify the important role that symmetry plays in the task of human scene perception. In the second part of the talk, I will review our more recent efforts to leverage these findings in human vision to improve the performance of a deep learning computer vision system addressing the same task. This is part of a new research program that seeks to draw on human vision to better inform the design of computer vision systems.
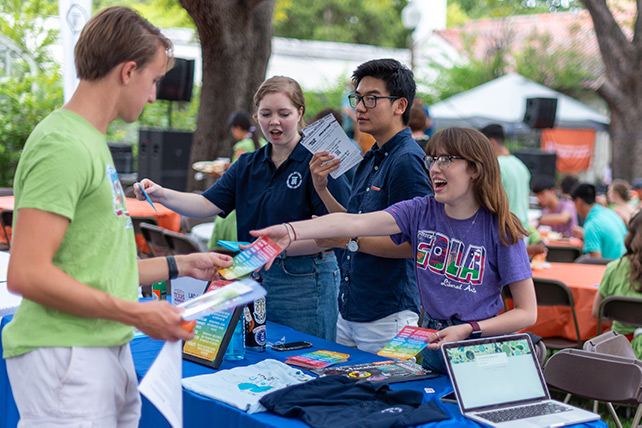
David Freedman
Primate Oculomotor Networks are Recruited by Abstract Cognition
Humans and other animals are adept at learning to perform cognitively-demanding behavioral tasks. Neurophysiological recordings in non-human primates during such tasks find that task-related cognitive variables are encoded across a wide network of brain regions, but particularly strongly so in core oculomotor brain regions such as the frontal eye field, superior colliculus, and posterior parietal cortex--even in tasks which require gaze fixation and in which monkeys indicate their decision with hand, rather than eye, movements. This talk will discuss the causal significance of the observed cognitive encoding in oculomotor circuits, as well as new evidence for cognitively modulated incidental gaze shifts akin to a "poker tell" observed in human subjects.
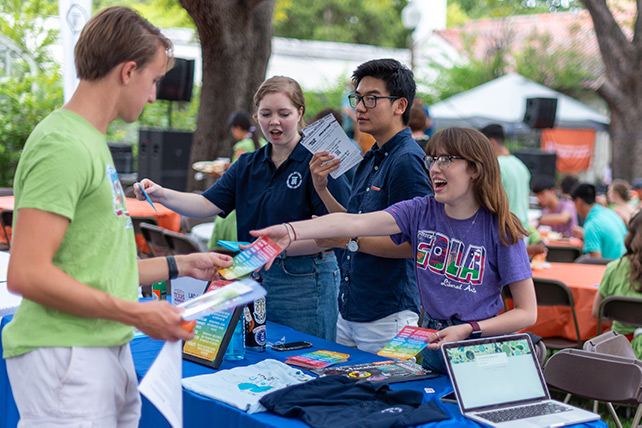
Avniel Ghuman
Neural encoding of real world face perception
How does your brain represent your daughter’s face, its expressions and movements while you are playing a board game together? This question illustrates a central goal of neuroscience – to understand how the brain processes information during natural behavior in the real world. Controlled laboratory experiments have led to important discoveries, such as the existence of an extended face processing network and aspects of how it codes for faces. However, the fundamental question of how our brains process the expressions and movements of real faces during natural, real world interactions with other people remains open. Addressing this central neuroscientific goal requires answering two intertwined questions: Can we model the unconstrained variability of faces during free, natural social interactions in the real world? And, if so, can we test hypotheses about neural representations to understand neural tuning for facial expressions and motion during interactions in the real world? We investigated the neural basis of real-world face perception using multi-electrode intracranial recordings in humans during unscripted interactions with friends, family, and others. Computational models reconstructed the faces participants looked at during social interactions, including facial expressions and motion, from brain activity alone. The results revealed neural tuning that highlighted a critical role for the social-vision pathway, a network of areas across parietal, temporal, and occipital cortex. The brain was more sharply tuned to subtle expressions over strong ones, which was confirmed with controlled psychophysical experiments. The results also emphasized the critical role of saccades in organizing face processing in the brain during natural vision. These findings revealed the human social vision pathway encodes facial expressions and motion as deviations from a neutral expression prototype during natural social interactions in real life.
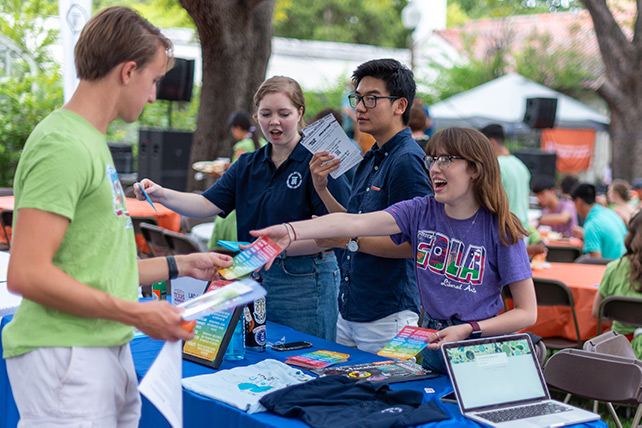
Liberty Hamilton
Modulation of neural responses during self-generated speech using intracranial recordings
Prior work on speech processing in the brain using intracranial recordings has shown that the superior temporal gyrus (STG) can be separated into two subregions: one posteriorly that encodes acoustic onsets, and one more anteriorly that evokes a more sustained response. The onset responses in the pSTG appear to be important for segmenting continuous speech information, but it is unclear how these responses are modulated by audiomotor feedback during speech production -- for example, when people hear their own voice versus external speech. In this talk, I will describe our findings from intracranial recordings from 17 patient participants across a wide age range (aged 8-37) while they performed a dual speaking and listening task and intracranial signals were recorded from auditory, motor, prefrontal, and insular regions of the brain. Participants read sentences aloud and then heard either immediate playback of the same sentence they had said, or playback of another sentence they had uttered in a previous trial. Overall, we found strong, specific suppression of neural onset responses in the STG that were not related to the predictability of playback. In addition, we found a specific subregion of the insula that exhibited fast latency dual onset responses during both perception and production. Our results have implications for understanding audiomotor feedback and the interactions between naturalistic speech perception and production.
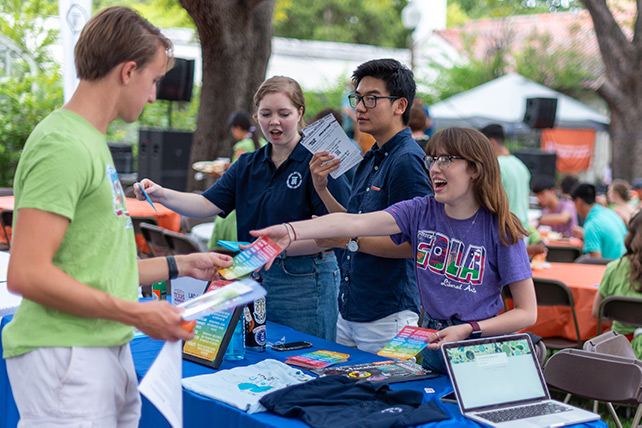
Ben Hayden
Neuronal basis of syntax and semantics in natural speech
The ability to record responses of single neurons in awake humans allows us to understand the neurocomputational foundations of language during natural speech. We recorded responses of neural populations in hippocampus and anterior cingulate cortex during both speech listening and conversations. We find that single neurons in both regions use a dense code with mixed selectivity to encode both speaker identity and word meanings. Meanwhile we find that morphosyntactic processes correspond to specific and consistent vectorial transformations.
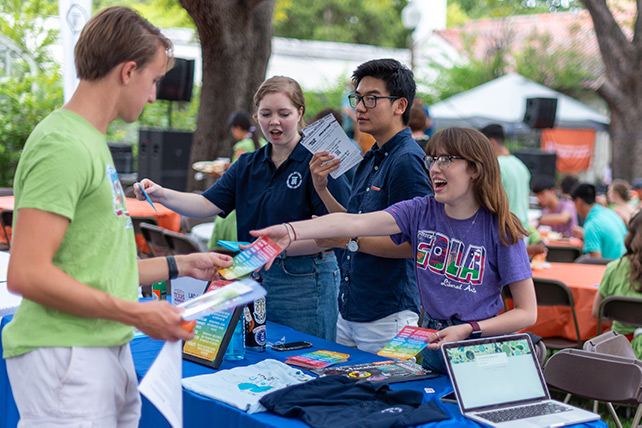
Kohitij Kar
From independent snapshots to integrated streams: Probing Neural Mechanisms of Dynamic Scene Perception in the Primate Brain
How does the brain represent, predict, and interpret the dynamic visual world? While much of our understanding of visual object processing has been shaped by studies conducted with static images, recent work has moved beyond these constraints to investigate the same fundamental processes in dynamic contexts. In this talk, I will first review key advances we have made using static image paradigms to develop sensory computable, mechanistic, anatomically referenced, and testable (SMART) models of object recognition, thereby elucidating the underlying neural mechanisms. I will then highlight our ongoing shift toward dynamic scene perception, encompassing various facets of scene dynamics — object motion, facial motion during emotion transitions, action prediction and the role of motion vs. appearance information in those paradigms, future scene outcome predictions with limited dynamic content, and the intricate interplay of form and motion revealed by camouflage-breaking tasks. Alongside a discussion of how we evaluate dynamic neural signals and computational models, I will touch on our use of non-human primate behavioral testing (including chemogenetics) and outline future directions in this rapidly evolving research landscape. This integrative approach aims to deepen our understanding of how the visual system—and the computational models used to operationalize our understanding of it—effectively handles dynamic, real-world scenarios.
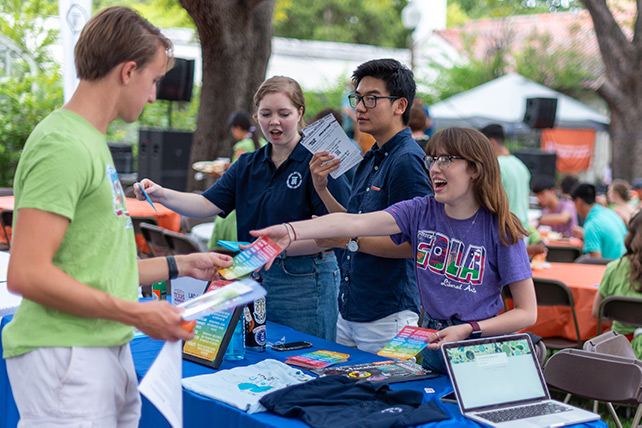
Julio Martinez-Trujillo
Why do primates have view cells instead of place cells?
Hippocampal place cells, which encode an individual's spatial location during navigation, have been widely reported in rodent species such as rats and mice. However, studies in primates have instead identified hippocampal cells that encode views of the environment. We investigated spatial navigation in two primate species, macaques using virtual reality, and freely moving marmosets. We found that their navigation strategies differ from those of rodents. Moreover, we observed a predominance of neurons in the CA1 and CA3 subfields of the hippocampus that encode views of the environment, as well as other variables related to gaze direction and head kinematics. We propose that the evolution of a visual system adapted for daylight navigation has shaped spatial navigation strategies and their neural substrates in the primate hippocampus.
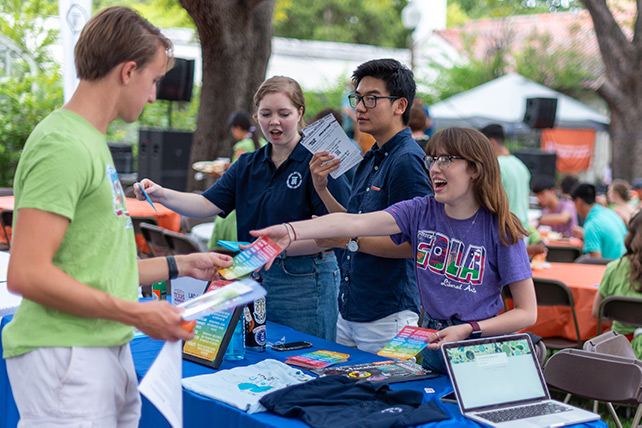
Jude Mitchell
Neural mechanisms of active foveal vision in marmoset monkeys
Primates use high acuity central vision to scan visual scenes and monitor distant objects. Each saccadic eye movement brings objects of interest to the fovea, a central region within 1 visual degree eccentricity of highest acuity. Despite its importance in primate vision, few studies have examined foveal representations in visual cortex due to technical challenges. Here I present advances from my laboratory in recording foveal neuron activity as marmoset monkeys actively forage visual scenes and moving targets. First, we have used high-resolution eye tracking during free-viewing of video displays to correct for instantaneous eye position and map foveal visual receptive fields in V1 (Yates et al., Nature Communications, 2023). This free-viewing approach has also allowed us to examine how eye movements modulate visual signals. We find that each eye movement initiates a wave of suppression followed by rebound activity that has distinct timing across the population, and could support a coarse-to-fine processing strategy (Parker et al., Nature Neuroscience, 2023). These saccade-related modulations can also carry top-down predictions important for remapping attention during saccades. In a second experiment, we recorded from peripheral and foveal representations in extra-striate areas MT/MTC while marmosets made saccades to moving targets. Peripheral neurons exhibited gain enhancement when the motion stimulus in their receptive field was the target of an upcoming saccade, consistent with pre-saccadic attention (Coop et al., J. Neuroscience, 2024). In addition, a subset of neurons with foveal receptive fields also showed pre-saccadic enhancements specific to the target. This was possible because those neurons had receptive fields that extended out from the fovea into the periphery and could respond when the peripheral stimulus was the target of the saccade. Those enhancements had spread to the other foveal neurons after the saccade, including neurons in the opposite visual hemi-field. This resulted in enhanced processing for the target anticipated at the fovea. These mechanisms are ideal to support continued selection of the target as it is tracked across eye movements.
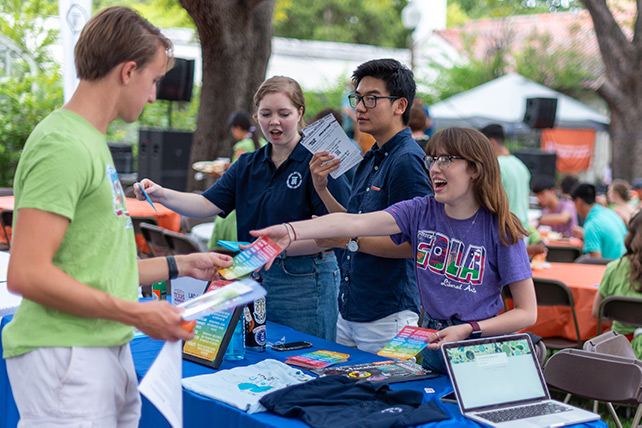
Emily Oby
Structure and flexibility of neural population activity
Learning is a critical part of life. We learn to walk, to communicate, and to reason with our world. Some behaviors are learned quickly. Other behaviors are much more difficult to learn, requiring weeks of effort and the guidance of a coach. I use brain-computer interfaces (BCIs) to examine how neural population activity changes with learning. First, I will show that in a BCI learning task, the structure of neural population activity constrains what is learned on short time scales. It takes many days and an incremental training procedure to change that structure and generate new patterns of neural activity. Then, I will show that neural population activity is also temporally constrained. Animals were unable to violate the naturally-occurring temporal structure in neural population activity observed in motor cortex when directly challenged to do so. These results provide empirical support for the view that activity time courses observed in the brain indeed reflect the underlying network-level computational mechanisms that they are believed to implement.
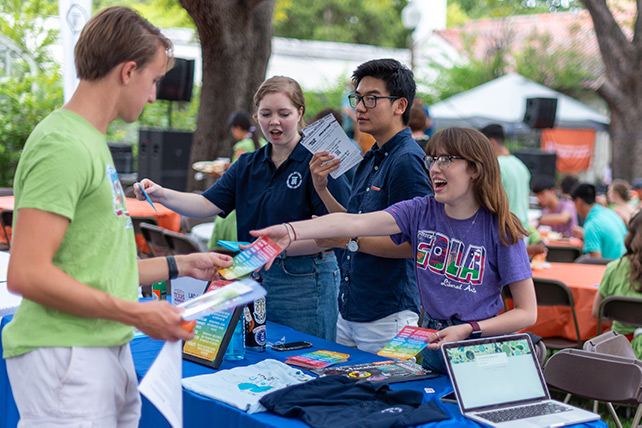
Constantin Rothkopf
Computational elements of goal-directed sensorimotor behavior
Goal-directed sensorimotor behavior in natural tasks reveals the intricate relationship between perception, memory, cognition, decision-making, planning, action selection, and learning. In this talk, I will present several naturalistic tasks that elicit these behaviors together with a unified account of these processes based on Partially Observable Markov Decision Processes (POMDP). For example, goal-directed navigation requires continuously integrating uncertain self-motion and landmark cues into an internal sense of location and direction, concurrently planning future paths, and sequentially executing motor actions. As humans navigate, they actively learn the structure of their environment, constructing an internal model or map that guides where and how they seek information. This active learning process subsequently shapes active perception, enabling individuals to reduce uncertainty about their position in space through targeted eye, head, and body movements. A POMDP model of probabilistic path planning, specifically optimal feedback control under uncertainty gives rise to diverse human navigational strategies previously believed to be distinct behaviors and predicts quantitatively both the errors and the variability of navigation across numerous experiments. This furthermore explains how sequential egocentric landmark observations form an uncertain allocentric cognitive map, how this internal map is used both in route planning and during execution of movements, and reconciles seemingly contradictory results about cue-integration behavior in navigation. The results show that humans coordinate their eye, head, and body movements to actively shape their spatial uncertainties, highlighting the intertwined roles of active learning and active sensing in human spatial navigation. Taken together, the talk will present a parsimonious explanation of how patterns of human goal-directed sensorimotor behavior arise from the continuous and dynamic interactions of uncertainties in perception, cognition, and action.
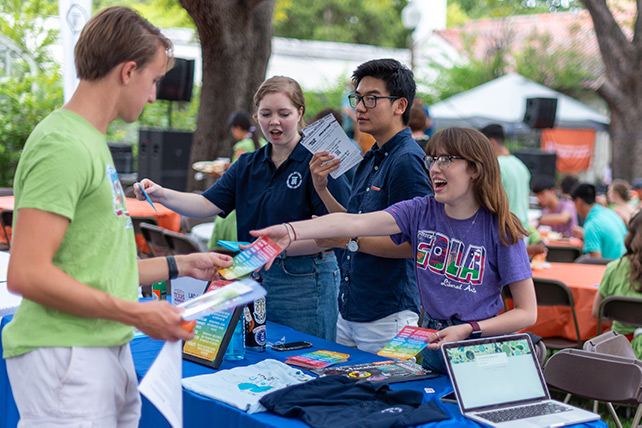
Andreas Tolias
Foundation models of the brain
You … your memories and ambitions, your sense of personal identity and free will, are in fact no more than the behavior of a vast assembly of nerve cells …’ Crick’s words capture the profound challenge of decrypting the neural code. This challenge has long been hindered by our limited ability to record activity from large neuronal populations under the complex, variable conditions in which brains evolve, and our capacity to model the intricate relationships between stimuli, behaviors, and neural activity.Recent breakthroughs are starting to overcome these barriers. Cutting-edge technologies now enable large-scale recordings, while AI can construct predictive brain models that link stimuli, neural activity, and behavior. These digital twins open the door to limitless in silico experiments, testing theories that are otherwise impossible at scale in living brains. I will discuss our work in creating these digital twins and uncovering neural representation mechanisms, which we validate with closed-loop experiments.
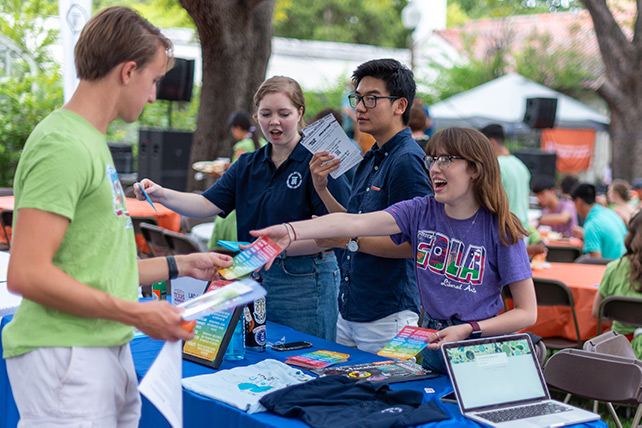
Xue-Xin Wei
Normative models of natural tasks
Neural systems adapt to the structure of the environment and task demands to support behavior. My lab uses a normative approach to study such adaptation. In this talk, I will first present a principled framework that links the structure of the neural representation to behavior in various psychophysical tasks. Next, I will show that optimization-based recurrent neural networks can be used to study how neural circuits perform cognitive computation efficiently. Together, this research leads to insights into the questions of (i) how the environmental statistics and task demands determine the neural computations, and (ii) how these computations support behavior.
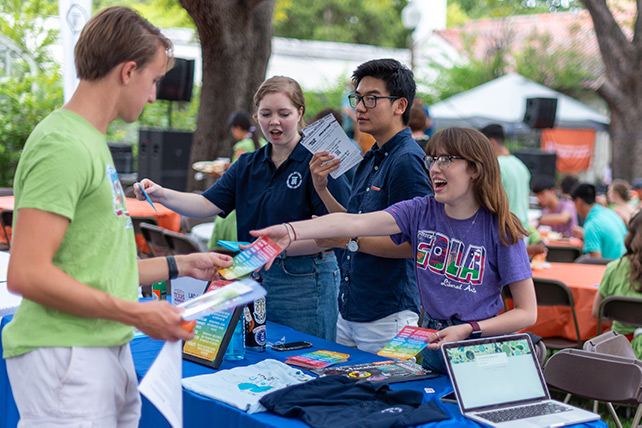