Foundations of Data Science I
This course lays the foundation for data science education targeting psychological and brain science students. No previous coding experience is required. The students will be introduced to basic concepts and tools for data analysis. The focus is on hands-on practice and enjoyable learning. The course will use python as the programming language, and Jupyter Notebooks as the development environment (our “home base”) for the examples, tutorials, and assignments. We use Jupyterlab Notebooks because they are both the industry standard and a nice way to load, visualize, and analyze data as well as describe our findings in one environment. We will also learn GitHub to document changes and backup our work and, eventually, for use as a collaboration tool. The courseis flipped, meaning that the students will be required to follow short pre-recorded video lectures and or written tutorials, and then we’ll spend the class time actually writing code and playing with data. Hands-on data analysis, final projects and associated presentations will be mandatory for the completion of the course. The final outcome for the class is that each student will have a GitHub repository with all of their work (Jupyter notebooks, data, etc.), including a final project that will be presented to the class.
Specific topics to be covered include: GitHub, Jupyter Notebooks, Python Programming, Data Visualization, Simulation and Data analysis, Data Modelling, Ordinary Least-square regression and Generalized Linear Models.
Course Prerequisites: There are no prerequisites for the course. Be sure to install the following software on your laptop:
○ Python installation via Anaconda: https://www.anaconda.com/products/individual
○The gitcode management too: https://git-scm.com/
LEARNING ACTIVITIES
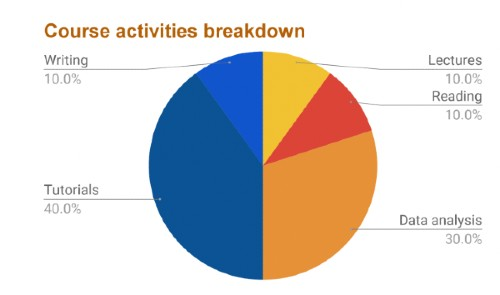
This caption describes the image above.
This class will comprise various learning activities. A. In-class coding exercises to walk through the coding and data analysis tools. B. Papers, book chapters, videos, and notes will be required as reading in preparation for some classes. C. Project-driven data analysis students will complete a series of reports to practice the coding and data concepts covered by the tutorials. The course is flipped, meaning that students will be required to read tutorials and readings before coming to class. The time in class will be spent working on the tutorials, coding examples and data analysis tasks. The time spent on the class can be subdivided approximately as described by the graph to the left. Students are expected to learn to use code written for mixed software environments, github.com, Linux/Unix, and programming languages such as Shell commands, and Python. Success in the most exciting research projects and jobs, either in Academia or Industry, requires mastering complex emotional, social and technical skills. The most challenging and interesting jobs require the flexibility to quickly master multiple types of theoretical understanding and technologies. This course will teach not only about coding but also will allow practicing working in a project-oriented manner using modern data management and analysis tools.
STUDENT PERFORMANCE EVALUATION
- Final project. A data analysis project report with text and figures describing the goals and results. A comprehensive GitHub repository with Jupyter notebooks to generate figures and analyses (15%).
- Four data science reports. There will be 4 reports in total due roughly every four weeks (15% each -60%): A. GitHub and Shell. B. Data Simulation. C. Data Modelling. D. Data Analysis.
- GitHub status. The engagement of the students on GitHub for the repositories relevant to each project and report will be evaluated using GitHub’s metrics (10%).
- Attendance. Come to class virtual or not (10%).
- Coding Engagement. Students will submit each class’ notebook on Canvas as PDFs (Tuesdays and Thursdays by midnight, 5%).
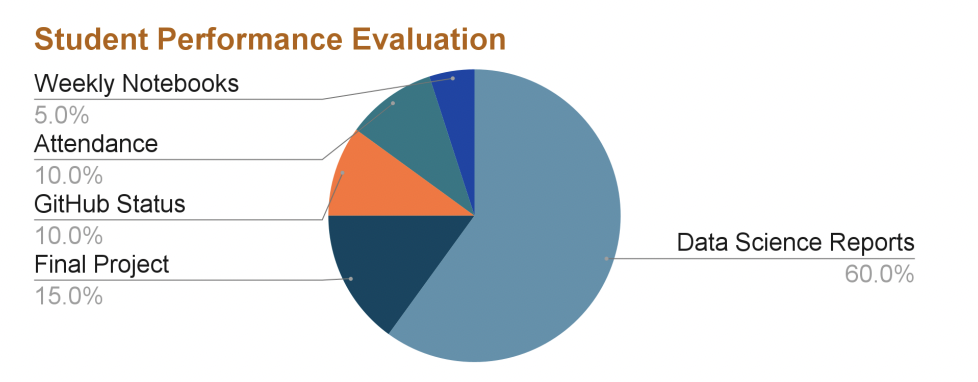
This caption describes the image above.